Generating knowledge is not the ultimate aim of a Learning Health System. Rather, the goal is to continuously improve health and care. For this to happen, the knowledge generated in the previous steps must be translated into action or mobilised. This section outlines how knowledge within a Learning Health System can be represented in a machine-readable format and applied at the front line.
Representing and managing knowledge
A Learning Health System can generate knowledge, but it must also integrate knowledge generated beyond the system: in previous studies, for example. Often knowledge is represented in books, journal articles, clinical guidelines and protocols. It can be passed on through formal and informal education or training. It can be used to redesign care environments and health interventions. These processes are often slow. These will all continue to be valuable ways of representing knowledge within a Learning Health System, but in order to scale-up and speed-up the process of knowledge improving practice, knowledge must be consumable by the computer systems in use at the frontline.
NHS Library and Knowledge Services
In England, the national NHS Library and Knowledge Services team procures a clinical decision support tool for all NHS staff and learners, enabling seamless access to a high-quality digital knowledge resource. It has supported knowledge management within the health service, providing a toolkit that covers traditional and digital forms of knowledge [86]. It offers e-learning on the [184] a suite of techniques through which users can articulate and share their learning from experience. Library and Knowledge Services in general are becoming more, rather than less, important within Learning Health Systems [185]. The English service has been shown to return significant value.
There have been efforts to standardise the representation of knowledge so that it can be applied at scale within a Learning Health System. OpenClinical.net, a collaboration between University College London and Oxford University, maintains an open-access and open-source repository of medical knowledge in a machine-readable format. OpenClinical uses the PROforma process modelling language to create machine-readable clinical guidelines, clinical algorithms, etc [39].
A more recent example is provided by Mobilizing Computable Biomedical Knowledge (MCBK), a new international community led by the Department of Learning Health Sciences at the University of Michigan. The group’s goal is to ensure that biomedical knowledge in computable form is Findable, Accessible, Interoperable and Reusable (FAIR). MCBK has formed multidisciplinary workgroups to develop key aspects of the solution:
- Standards
- Technical Infrastructure
- Trust & Policy
- Sustainability & Inclusion
Once knowledge is computable, processes can be automated; it can be fed into decision support systems to help guide decisions, or it can be used to assess the
delivery of care against the knowledge base. These uses reduce the time taken for new knowledge to improve care, compared to the traditional system, which relies on the knowledge being published in a journal, read, acted upon and audited.
The Learning Healthcare Project collaborated with the Faculty of Clinical Informatics, NICE, the British Computer Society, HDR UK and HL7 UK to run a workshop on Mobilising Computable Biomedical Knowledge, inspired by the global MCBK community [27]. The knowledge generated by the workshop is available in traditional forms, including video recordings [96] and as a series of seven journal articles, forming a special issue of BMJ Health & Care Informatics [97].
National Institute for Health and Care Excellence
The National Institute for Health and Care Excellence (NICE) [186] produces knowledge in the form of evidence-based guidance to the English NHS. This knowledge is based on evidence developed through a range of scientific methods [187] and interpreted through a deliberative committee process [186]. Figure C shows the range of guidance produced over the last 20 years.
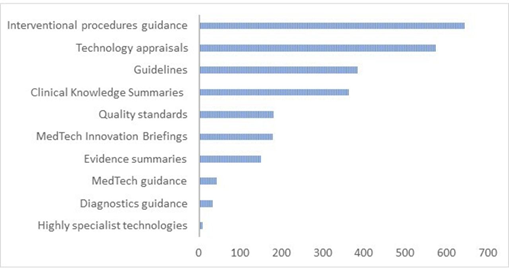
The MCBK workshop explored NICE’s ambition to broaden its scope from single condition, narrative guidelines to structured recommendations encompassing multi-morbidity, polypharmacy and wider health system considerations [189].
The NICE team have considered their current products against the AHRQ 4 levels of knowledge (Figure D). While currently the products are primarily narrative, there is an ambition to make their recommendations – and the evidence that underpins them – more structured, to aid maintenance and surveillance. Although NICE has explored the potential for reverse engineering structured guidance from previously generated narrative guidance, the group has found it to be inefficient and potentially unsafe. Instead, NICE considers a co-production model to be a better way of bringing together the guideline development committee and those who produce the executable guidance.
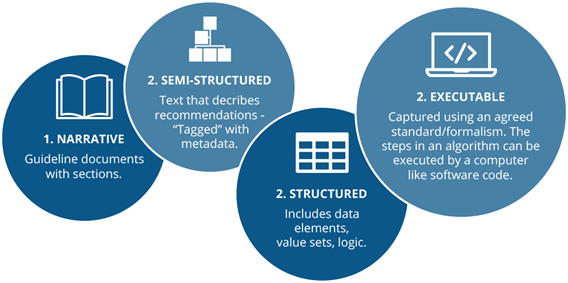
NICE has recognised that a move to more structured or even executable knowledge products will require a change in processes, methods, technologies, and skills, which cannot be achieved quickly. NICE is considering which existing formalisms, coding and information standards could be used to represent their knowledge. They are also considering issues around liability when something goes wrong, for those who develop guidance, those who structure it and those who apply it. This is further complicated when knowledge is disseminated through third-party decision support systems.
The lack of existing large exemplars means there is limited evidence to show that investment in resolving these challenges would result in a greater uptake of guidelines and improved outcomes for patients. NICE is attempting to resolve this gap through its NICE Connect transformation programme [31].
Challenges for Medical Knowledge Providers
In a session led by BMJ Best Practice, the MCBK workshop explored the challenges that Medical Knowledge Providers face in getting knowledge into practice [41, 98]. This was supplemented by a session focusing on the associated technical [98] challenges [99, 100]. These sessions highlighted seven challenges that should be considered by any organisation seeking to mobilise computable biomedical knowledge:
- Size of the task: BMJ Best Practice covers over 1,000 topics, while NICE [41] has published over 2,000 products. Maintaining these catalogues alone is a huge undertaking, yet they represent only a very small fraction of total medical knowledge.
- Standards: These are necessary to make knowledge interoperable between systems. SMART on FHIR (for building Apps on health platforms) [101], Guideline Interchange Format [102], CDS Hooks (for clinical decision support) [103], and openEHR (for EHRs) [104] are in use, along with a range of other open and proprietary standards.
- Making knowledge computable: This is known as knowledge formalisation. All background knowledge is made explicit, along with the common sense required for interpretation. Meanwhile, ambiguity is removed, enabling interpretation by a machine [99]. This is a highly skilled process with scope for error and harm to patients.
- Inference: To use computable knowledge in areas like clinical decision support, it must be manipulated to produce actionable outputs. This process is called inference. Logical or probabilistic reasoning combines knowledge, such as a guideline, with specific data about individual patients. For example, does the patient require a CT Head? This reasoning can exploit IF-THEN rules (eg IF patient meets any of these criteria THEN perform CT Head). It becomes much more difficult if there are multiple conflicting knowledge sources, multi-morbidities or if the accuracy of the knowledge is uncertain.
- Outcomes: As seen in the NICE example (See NICE Box), there is a need to trace the ways that computable knowledge impacts on patient outcomes as well as process measures, in order to justify investment and improve systems.
- Safety: Computable knowledge that is intended to be applied at the point of care can cause harm. In England, compliance with clinical safety standards DCB0129 (for manufacturers) [105] and DCB0160 (for deploying organisations) [106] is mandatory under the Health and Social Care Act 2012 [107]. Under the Medical Devices Regulation published by the European Commission in 2017, software such as a clinical decision support system would be classed as a Medical Device [108], so would need to be registered with the Medicines and Healthcare Products Regulatory Agency (MHRA) and audited by a notified body [109].
- Professionalism: Clinicians need problem solving and procedural skills, healthy attitudes and professional behaviours, which cannot be replaced by computable knowledge. It is important to recognise the limits of such systems.
Progress on Mobilising Computable Biomedical Knowledge
Despite much technological progress, the goal of Findable, Accessible, Interoperable and Reusable (FAIR) [110] knowledge has still not been widely achieved. Much knowledge is not computable, as it resides in books, articles, PDFs etc that are proliferating faster than clinicians can consume.
Most computable knowledge is in formats that lack common standards, such as proprietary decision support systems. Systems rarely address the real-world complexity of multimorbidity, polypharmacy, resource constraints etc. This limits the development of Learning Health Systems and causes patient safety issues, particularly when knowledge is encoded within systems that cannot be readily evaluated [111]. When such systems malfunction, large numbers of patients can be impacted [112].
Those developing computable biomedical knowledge can benefit from the open-source tools, infrastructure and repositories developed by MCBK and others [38, 113]. They can also publish their knowledge objects for peer review and reuse [114].
It is important to note that in practice, the evidence applied to decisions [115] does not necessarily comprise the most “scientific” or objective items. Decision makers often prefer local knowledge and the experience of near neighbours. This judgement can be difficult to codify in a computerised system. It may be sensible for an organisation to start computerising knowledge in areas that are characterised by the most agreement and least complexity; however, the richest opportunities may lie beyond.
Scottish National Decision Support Programme
This workshop explored a new Scottish programme to mobilise knowledge through decision support systems [35, 36]. It is aligned to the broader national health strategy and focuses on improving outcomes in key areas. Each decision support tool is developed through a participatory co-design process, engaging all stakeholders. It has developed a technology architecture to facilitate joined-up development across partners in health, academia and industry (Figure E), which could be applied elsewhere.
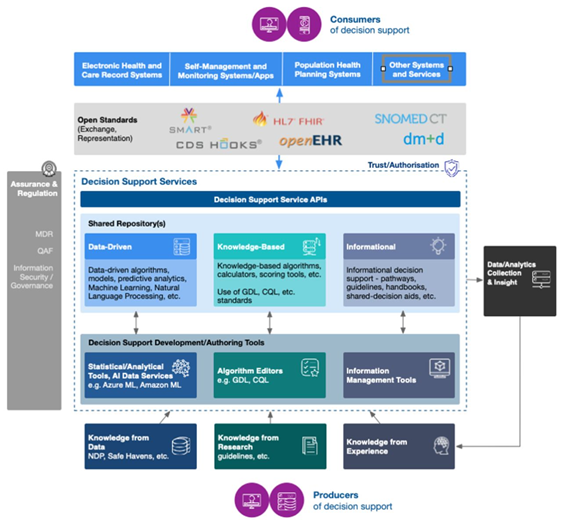