Learning Health Systems could revolutionise healthcare practice. They have the potential to enable personalised, proactive services, capturing and analysing clinical data that can continuously inform and improve health decision making and practice [118, 119] . But six years after the first Learning Healthcare Project report [1] and 13 years since the IoM popularised the concept [120], no nation, region or individual healthcare provider has fully realised this promise. Previous sections have discussed the delivery of knowledge to the point of care. This is necessary, but more is needed to ensure this knowledge actually influences practice.
In the traditional “pipeline” model of healthcare innovation, basic research progresses to the prototype stage, before moving to Randomised Controlled Trial, publication and guideline production, resulting in a change in practice [121].
Early Learning Health System thinking recognised the limitations of this model. Randomised Controlled Trials could not answer all the clinical questions about the wide variety of real-world patients. Even when evidence existed, it was often not widely employed [122]. Learning Health Systems offered an improvement, showing how routinely collected data could provide answers to more questions and how digital systems could deliver that knowledge to the point of care. The pipeline became a cycle, but it maintained a stepwise nature.
Our original report covered a range of complex challenges in developing a Learning Health System, based on our study of international exemplars. These challenges included comorbidities, technology, interoperability, data quality, Information Governance, regulation, ethics, leadership, behaviour change, value, and patient, clinician and organisational acceptability [1].
Until this point, there has been no accepted framework for considering these challenges when planning, implementing and evaluating Learning Health Systems, with most effort so far spent on developing the technical aspects of the systems. Consequently, we have not seen widespread adoption, scale-up and spread of Learning Health Systems.
Complexity in the Learning Health System
Healthcare is increasingly recognised as a complex adaptive system [123]. Snowden [124] outlined the characteristics of a complex system:
- A large number of interacting elements
- Non-linear interaction – minor changes can produce disproportionate consequences
- Emergence – the whole is greater than the sum of its parts
- Elements evolve with one another and with the environment in an irreversible way
- While it may appear predictable in retrospect, this is misleading: external conditions and the system are constantly changing
- Agents and the system constrain one another over time, unlike an ordered system (where the system constrains the agent) or a chaotic system (no constraints)
The Cynefin Framework [125] can be used to understand whether a given system is Obvious/Simple, Complicated, Complex,
Chaotic or Disordered. This understanding can suggest the most appropriate way to intervene (Figure F):
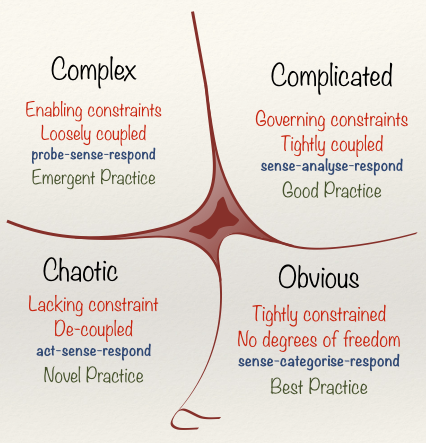
Simple Context: These are process-oriented systems in which there is clear cause and effect and best practice can be established. The solution is obvious to everyone. For example, a system for allocating patients to child or adult services: if their age is under 18, allocate to child services, and if their age is 18 or greater, allocate to adult services. A command-and-control approach can be effective in such systems, and extensive discussion is unnecessary.
Complicated Context: Cause and effect is still present, but it may not be obvious. There could be multiple right answers, and expertise is required to analyse the situation: for example, building or repairing a piece of medical equipment. Good practice is therefore more appropriate than best practice. With enough time, at least one right answer can usually be found.
Complex Context: Complex systems are in constant flux, so cause and effect break down. The whole is greater than the sum of its parts. Snowden uses the analogy of a Ferrari (complicated but static) and a rainforest (complex). While an expert can take the Ferrari apart and put it back together, a rainforest is more than the sum of its parts. Command and control management, fail-safe plans and defined outcomes do not work in complex contexts, due to their unpredictability. Instructive patterns emerge by setting up many parallel, safe to fail experiments, and a way forward can be determined by amplifying interventions that work and dampening those that do not [126]. Learning Health Systems generally fall into this complex category.
Chaotic Context: This is a crisis situation. The relationship between cause and effect is impossible to determine and constantly changing. Searching for the right answer is futile and immediate action is required to establish stability, allowing the situation to move from chaotic to complex. A directive leadership approach is required, but this is also a time when innovation can be accelerated; hence the term, “never let a good crisis go to waste”.
Disordered Context: It is not clear which context prevails, and there are multiple perspectives. Resolution lies in breaking the situation down into its constituent parts, each of which can be assigned to one of the other contexts.
Understanding which context describes a particular situation can help determine the most likely successful approaches. Situations can also shift between contexts. For example, a complex situation can become complicated when it is better understood, or a simple situation can become chaotic as a result of complacency.
For some experts, uncontrolled complexity explains the failure of many health IT interventions, including those associated with Learning Health Systems [127]. Complex systems may require alternative, more human approaches to governance and improving outcomes [128].
In 2017, Greenhalgh et al. [127] reviewed 28 technology implementation frameworks and integrated the findings with 400 hours of ethnographic observation and 165 semi-structured interviews. They used this to develop the Nonadoption, Abandonment, Scale-up, Spread, and Sustainability (NASSS) Framework for Health and Care Technologies.
The NASSS Framework identifies a range of challenges across seven domains, each of which can be
classified as simple, complicated or complex, in line with the Cynefin Framework [124]
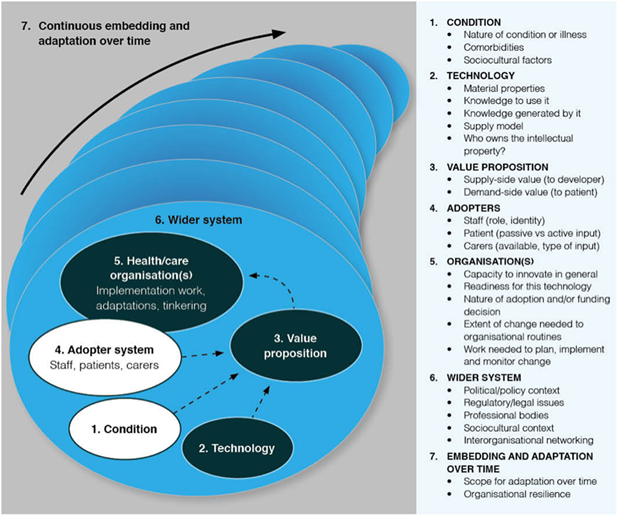
An expert workshop was held to inform this report, chaired by Prof Greenhalgh and attended by a group of experts in Learning Health Systems and implementation science [129].
The workshop considered how the NASSS Framework could be applied to Learning Health Systems, using four exemplars (See boxes below).
HealthTracker
A Clinical Decision Support Tool which incorporates ten different clinical practice guidelines into a single on-screen algorithm on the clinician’s desktop. It provides estimates of cardiovascular risk and suggests further investigations and lifestyle changes. The tool was deployed in 60 general practices in Australia. A series of evaluations showed some positive impacts from deployment, but also wide variations in the tool’s use between clinicians and practices[24].
PatientsLikeMe
PatientsLikeMe [34] is a US-based web platform that allows patients around the world to share their health-related experiences and outcomes in a highly structured format. It was established by brothers Jamie and Ben Heywood after another brother, Stephen, was diagnosed with Amyotrophic Lateral Sclerosis (ALS). Since 2011, patients have been able to record their experiences of living with a large range of other conditions. The platform now has 750,000 members. The data can be used by individual patients or researchers to learn from the experience of each patient. This data has also been linked to biological data and used to train AI algorithms. Recent studies have shown the platform’s peer-peer connections improve self-management and self-efficacy for users.
Evelina London – Children and Young People’s Health Partnership (CYPHP)
The Evelina London CYPHP model [22] provides coordinated and tailored care for children and young people that is responsive to their needs. It integrates primary and secondary physical health, mental health, social care and education through Multidisciplinary Team meetings. Anticipatory care is enabled by using a population health model that allows for early identification and intervention through primary and secondary care data systems. The model combines routine health administration data with patient-generated data via a portal, giving children’s health teams the information to plan care that is appropriate to a child’s physical, mental, and social needs. Templates embedded in electronic health records, guidelines, and decision support tools promote evidence-based care and systematic collection of data on quality of care and outcomes. The data generated is combined with other evidence to enable continuous improvement in a Learning Health System.
The Evelina London CYPHP model covers two inner-city boroughs of South London (Lambeth and Southwark) with poor child health outcomes and high Emergency Department attendance rates for children and young people, emergency hospital admission and hospital appointment use. The Evelina London CYPHP model is being implemented across general practices, schools and hospitals.
TRANSFoRm
The TRANSFoRm project [37] developed technology to enable a rapid Learning Healthcare System that improves patient care by speeding up translational research, enabling more cost-effective Randomised Control Trials and by deploying diagnostic decision support. The project brought together a multidisciplinary consortium of 21 partner organisations from ten EU member states and was deployed on multiple EHR systems across several countries.
The following sections outline how each domain (Figure F) can be applied to understand the level of complexity within a Learning Health System, illustrated by the examples above.
Subsections
[subpages]